Machine Learning Methods and Use Cases
- Ravendra Singh
- Nov 6, 2023
- 3 min read
Updated: Dec 21, 2023
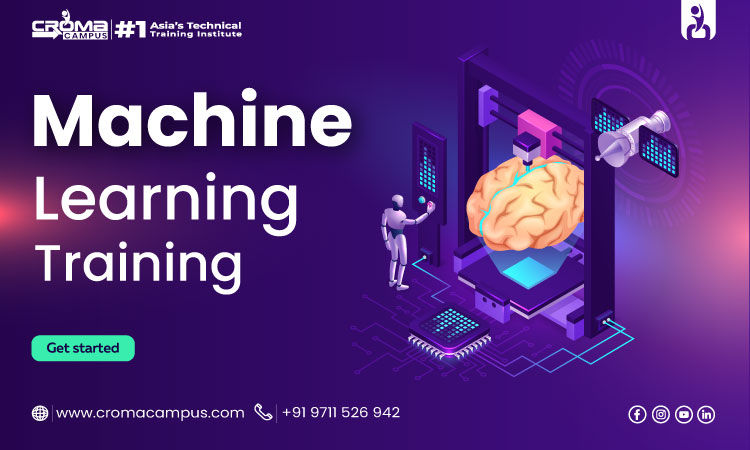
Machine learning, in short, ML, is an essential sub-field of Artificial Intelligence (AI). The ML models depend on data to learn and improve. Simply put, Machine Learning can be defined as the capability of modern machines to imitate human behavior. The ML models understand and build methods that leverage data to improve performance. Thus, the more an ML-integrated device receives data, the more it learns to perform different tasks. ML is a new and evolving concept. Therefore, one can join Machine Learning Course in Delhi to learn more about this technology and use this platform more effectively. Today, ML is used for personalized recommendations, checking spam mail, internet search engines, fraud detection, etc. Some popular job roles with ML training include Machine Learning Engineers, Machine Learning Developers, Data Scientists, NLP Scientists, etc. The average salary of ML professionals ranges from Rs. 3 LPA to Rs. 20 LPA in India.
Read this section to learn more about the important procedures followed in Machine Learning.
Important Machine Learning Methods
Machine Learning involves essential methods like Supervised Machine Learning, Unsupervised Machine Learning, and Semi-Supervised Learning.
Let us look at these ML methods in detail.
Supervised Machine Learning
Supervised learning is defined by the use of labeled datasets to train algorithms. It is used to classify data or predict outcomes accurately. Supervised ML helps organizations solve various real-world problems effortlessly.
Unsupervised Machine Learning
Unsupervised Machine Learning uses ML algorithms to analyze and cluster various unlabeled datasets. It discovers hidden patterns or data groupings without human intervention. Unsupervised ML is also used to reduce the number of features in a model.
Semi-Supervised Learning
Semi-supervised Learning offers a medium between the Supervised and Unsupervised Machine Learning. It uses a smaller labeled data set to guide classification and feature extraction from an unlabeled data set.
Use Cases In Machine Learning
Machine Learning models are used for a variety of purposes. It is an evolving technology that aims to improve the technical experience of the end users.
Let us look at the different use cases of ML in detail.
Speech Recognition: It is known as Computer Speech Recognition, Automatic Speech Recognition (ASR), or Speech-To-Text. It uses Natural Language Processing (NLP) to translate human speech into a written format.
Customer Service: Online chatbots answer frequently asked questions (FAQs) about shipping or provide personalized advice.
Computer Vision: This AI technology enables computers to derive meaningful information from digital images, videos, and other visual inputs and then take the appropriate action.
Recommendation Engines: AI algorithms can help to discover data trends that can be used to develop more effective cross-selling strategies.
Automated Stock Trading: AI-driven high-frequency trading platforms make thousands of daily trades without human intervention.
Fraud Detection: Banks and other financial institutions use ML to spot suspicious transactions and help organizations prevent potential fraud.
Conclusion
To sum up, Machine Learning (ML) is a sub-field of Artificial Intelligence (AI). It refers to the capability of modern machines to imitate human behavior. The ML models understand and build methods that leverage data to improve performance. Thus, the more an ML-integrated model receives data, the more it learns to perform certain tasks. Today, various Machine Learning Certification are used for internet searches, personalized recommendations, checking spam mail, fraud detection, etc. Furthermore, ML is extensively used for speech recognition, computer vision, and stock trading, and so on. This has generated huge demands for professionals skilled in various Machine Learning models. Some popular job roles with ML training include Machine Learning Engineers, Machine Learning Developers, Data Scientists, NLP Scientists, etc. Moreover, Machine Learning involves essential methods like Supervised Machine Learning, Unsupervised Machine Learning, and Semi-Supervised Learning.
Comments